Written by: admin
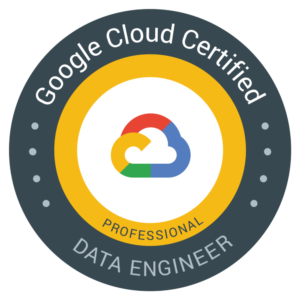
Overview
The Google Cloud Certified Professional Data Engineer certification is a globally recognized credential that honed your abilities in making data-driven choices by maximizing the acquired data. The certification programme effectively demonstrates how to gather, process, and analyses data in order to provide relevant insights. This thorough course intends to provide practical understanding of how to design, create, manage, and debug data processing systems, with an emphasis on the system’s critical elements such as dependability, scalability, fault-tolerance, fidelity, security, and efficiency.
A Data Engineer is responsible for performing data analysis to predict future business outcomes, developing statistical models to assist decision-making, and developing machine learning models to simplify and automate major business operations.
Why choose a Professional Data Engineer?
This data engineering certification programme will help you to:
- Data structures must be defined and managed.
- Work with data processing systems
- Data analysis and integration of pre-built ML services
- Create a procedure for optimizing and analyzing data.
- Understand and use data visualizations
- Recognize and execute security and compliance
Who should participate
- Are you a data engineer, data scientist, cloud architect, DevOps engineer, or any other cloud professional interested in learning or using machine learning and data engineering skills on Google Cloud Platform (GCP)?
- Are you a prospective student or an entry-level professional who understands the foundations of GCP? And do you have the Google-recommended 3+ years of industry experience, including at least 1+ year creating and managing GCP solutions?
- If so, you may enroll in this programme to learn all you need to know about designing, deploying, and operationalizing machine learning models, as well as building, monitoring, and migrating data-driven apps.
Certification for Google Cloud Certified Professional Data Engineer
Before proceeding to the exam details, the main question is why get certified as a Google Cloud Data Engineer. Cloud computing is the answer to this question.
In other words, cloud computing is a new trend that is here to stay. You can get a much clearer picture by referring to the points below:
- For starters, the computer industry has expanded significantly. This expansion is expected to continue for the next five years.
- Second, the amount of data being introduced is increasing at a faster rate. For example, 90 percent of data was created in the last two years.
- Third, the ability to transform data into usable information is becoming more complex, necessitating the acquisition of new skills and abilities.
- Finally, a data engineer clearly fits into this category, which encourages certification in the field.
The Exam’s Prerequisites
Because different exams have different requirements, it is necessary to understand Professional Data Engineer requirements. The following are the requirements for the specific exam:
- The ideal candidate will be scalable and efficient.
- He or she should be able to design and monitor data processing systems, with a focus on security.
- Above all, a data engineer should be able to leverage and train pre-existing machine learning models on a continuous basis.
For example, if you fail the certification test, you can retake it within 14 days.
Similarly, if you fail the second time, you must wait 60 days before taking the exam again.
Furthermore, if you do not pass the third attempt, you must wait a year before attempting again. Above all, you must pay each time you take an exam.
What Is the Purpose of a Professional Data Engineer?
The Professional Data Engineer, or as it’s also known, the Professional Data Engineer on Google Cloud Platform, like all tests, allows Google to examine a variety of subjects. Because they test on a range of subjects at random, understanding the bulk of Professional Data Engineer curriculum is necessary. Be aware that experience requirements are frequently in place because they have observed the average person and what is required.
Exam Format and Information
Exam for Google Professional Data Engineer
A Professional Data Engineer collects, transforms, and publishes data to allow data-driven decision making. A Data Engineer candidate should be able to design, implement, operationalize, protect, and monitor data processing systems with a focus on security and compliance, scalability and efficiency, dependability and fidelity, and flexibility and portability.
Exam Name | Google Cloud Certified Professional Data Engineer |
Exam Duration | 120 Minutes |
Exam Type | Multiple Choice Examination |
Passing Score | No Scoring Criteria |
Exam Fee | $200 |
Eligibility/Pre-Requisite | None |
Validity | 2 Years |
Exam Languages | English, Japanese, Spanish, Portuguese |
Choose Your Preferred Learning Mode
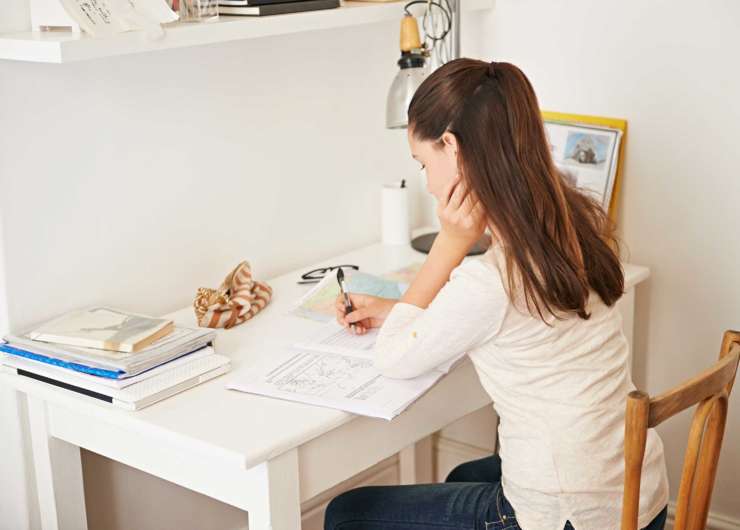
1-TO-1 TRAINING
Customized schedule Learn at your dedicated hour Instant clarification of doubt Guaranteed to run
ONLINE TRAINING
Flexibility, Convenience & Time Saving More Effective Learning Cost Savings
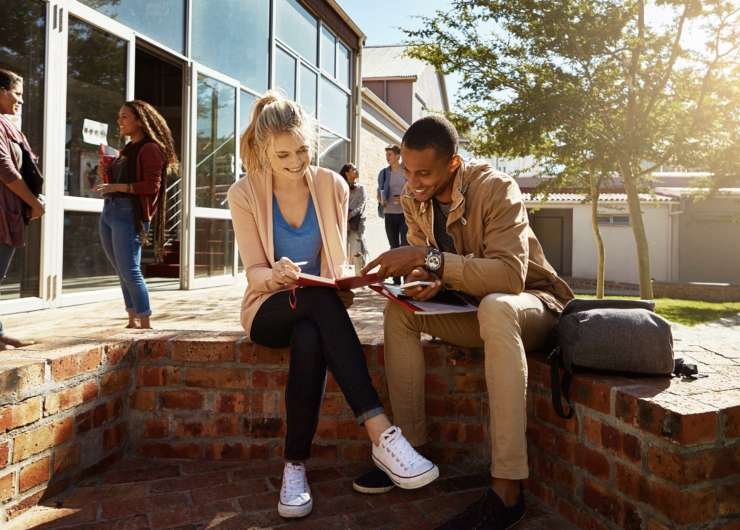
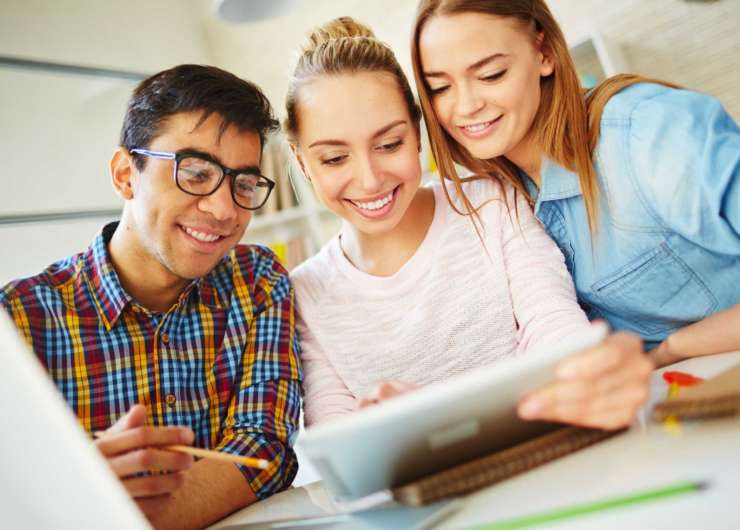
CORPORATE TRAINING
Anytime – Across The Globe Hire A Trainer At Your Own Pace Customized Corporate Training
Course Description
Let’s go over the exam outline after we’ve gotten a better understanding of the necessary details.
- First and foremost, appropriate storage technologies must be chosen.
- Following that, create designs for data pipelines.
- Third, create a data processing solution.
- Finally, data warehousing and data processing must be migrated.
- First and foremost, storage systems must be constructed and operationalized.
- Following that, pipelines with processing infrastructure will be built and operationalized.
- To begin, using pre-built ML models as a service.
- Then, an ML pipeline is deployed.
- Third, select the proper training and serving infrastructure.
- Finally, machine learning models must be measured, monitored, and troubleshooter.
- First, consider security and compliance.
- Then, with dependability and fidelity, ensure scalability and efficiency.
- Finally, ensure portability and flexibility.